DEA Land Cover (Landsat)
DEA Land Cover (Landsat)
Geoscience Australia Landsat Land Cover 25m
- Version:
1.0.0 (Latest)
- Product types:
Derivative, Raster
- Time span:
1988 – 2020
- Update frequency:
Yearly
- Next update due:
No updates planned
- Product ID:
ga_ls_landcover_class_cyear_2
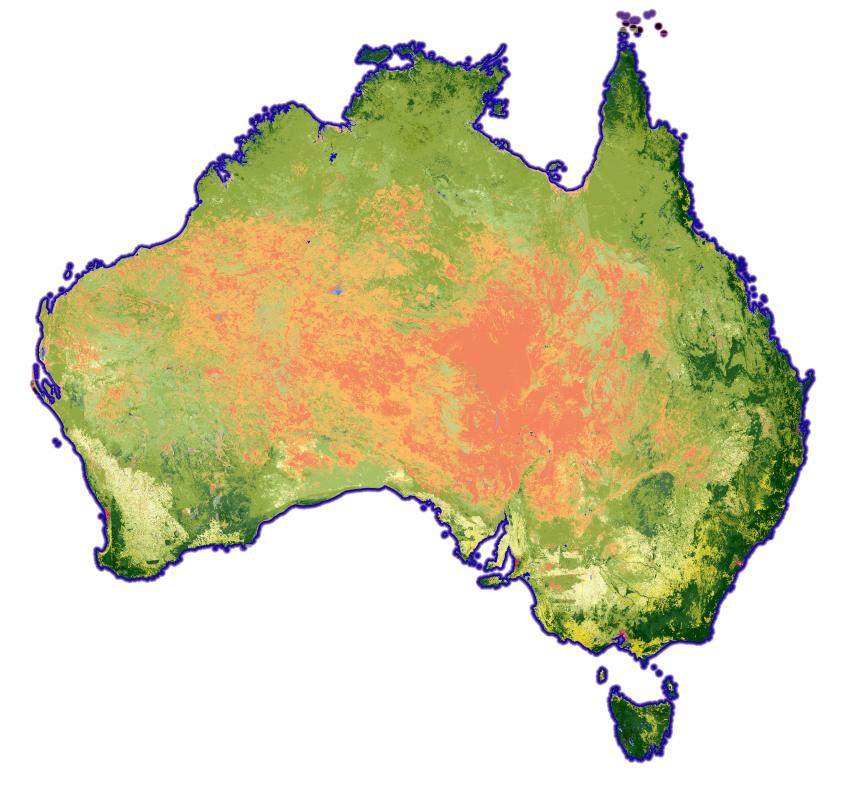
About
Digital Earth Australia (DEA) Land Cover translates over 30 years of satellite imagery into evidence of how Australia’s land, vegetation and waterbodies have changed over time.
New version in development
A new version of this product is being developed. Subscribe to the DEA newsletter to be notified of product releases.
Access the data
For help accessing the data, see the Access tab.
See it on a map
Data explorer
Access the data on AWS
Code examples
Web Services
Key details
Collection |
Geoscience Australia Landsat Collection 2 |
DOI |
|
Licence |
Cite this product
Data citation |
Tissott, B., Mueller, N., 2022. DEA Land Cover 25m. Geoscience Australia, Canberra. https://dx.doi.org/10.26186/146090
|
Paper citation |
Lucas, R., Mueller, N., Siggins, A., Owers, C., Clewley, D., Bunting, P., Kooymans, C., Tissott, B., Lewis, B., Lymburner, L., Metternicht, G., 2019. Land Cover Mapping using Digital Earth Australia. Data. 4(4):143. https://doi.org/10.3390/data4040143
|
Publications
Lucas R, Mueller N, Siggins A, Owers C, Clewley D, Bunting P, Kooymans C, Tissott B, Lewis B, Lymburner L, Metternicht G. Land Cover Mapping using Digital Earth Australia. Data. 2019; 4(4):143. https://doi.org/10.3390/data4040143
Christopher J. Owers, Richard M. Lucas, Daniel Clewley, Carole Planque, Suvarna Punalekar, Belle Tissott, Sean M. T. Chua, Pete Bunting, Norman Mueller & Graciela Metternicht (2021) Living Earth: Implementing national standardised land cover classification systems for Earth Observation in support of sustainable development, Big Earth Data, 5:3, 368-390, DOI: 10.1080/20964471.2021.1948179
Background
Land cover is the observed physical cover on the Earth’s surface including trees, shrubs, grasses, soils, exposed rocks, water bodies, plantations, crops and built structures. A consistent, Australia-wide land cover product helps understanding of how the different parts of the environment change and inter-relate. Earth observation data recorded over a period of time firstly allows the observation of the state of land cover at a specific time and secondly the way that land cover changes by comparison between times.
What this product offers
DEA Land Cover provides annual land cover classifications for Australia using the Food and Agriculture Organisation Land Cover Classification System taxonomy Version 2 (Di Gregorio and Jansen, 1998; 2005).
DEA Land Cover divides the landscape into six base land cover types, which are then further detailed through the addition of environmental descriptors. The structure is as follows:
Cultivated Terrestrial Vegetation
percentage of cover
life form (herbaceous only)
Natural Terrestrial Vegetation
percentage of cover
life form (woody or herbaceous)
Natural Aquatic Vegetation
percentage of cover
life form (woody or herbaceous)
water seasonality
Artificial Surfaces
Natural Bare
percentage of bare cover
Aquatic
water persistence
intertidal area
Applications
Annual Land Cover information can be used in a number of ways to support the monitoring and management of environments in Australia. These include, but are not limited to, the following areas in environmental monitoring, primary industries and the interests and safety of the Australian community:
Environmental monitoring
Ecosystem mapping
Carbon dynamics
Erosion management
Agriculture Sector
Monitoring crop responses to water availability
Understanding drought impact on vegetation
Community interests
Map urban expansion within Australia
Mapping impacts of natural disasters
Bushfire recovery
Technical information
DEA Land Cover is based on the globally applicable Food and Agriculture Organisation’s (FAO) Land Cover Classification System (LCCS) taxonomy Version 2 (Di Gregorio and Jansen, 1998; 2005). DEA Land Cover classifications have been generated by combining quantitative (continuous) or qualitative (thematic) environmental information (referred to as Essential Descriptors; EDs) derived from Landsat satellite sensor data. Several EDs have been generated previously by Geoscience Australia, including annual water summaries (Mueller et al., 2016), vegetation fractional cover (Scarth et al., 2010), mangrove extent (Lymburner et al., 2020) and the Inter Tidal Extent Model (ITEM; Sagar et al., 2017), whilst others have been developed more recently. These EDs have been combined to generate detailed, consistent and expandable annual classifications of Australia’s land cover from 1986 through to 2020.
DEA Land Cover consists of eight datasets: The base (level 3) classification, seven additional descriptor layers, and the final (level 4) classification combining the base classes with their associated descriptors.
Detailed layer descriptions, including known issues, are provided in the following sections.
Datasets, values and definitions
Level 3
The base Level 3 land cover classification
0: No data
111: Cultivated Terrestrial Vegetation (CTV)
112: (Semi-)Natural Terrestrial Vegetation (NTV)
124: Natural Aquatic Vegetation (NAV)
215: Artificial Surface (AS)
216: Natural Bare Surface (NS)
220: Water
Lifeform
Describes the detail of vegetated classes, separating woody from herbaceous
0: Not applicable (such as in water areas)
1: Woody (trees, shrubs)
2: Herbaceous (grasses, forbs)
Vegetation Cover
The measured cover of vegetated areas
0: Not applicable (such as in bare areas)
10: Closed (>65 %)
12: Open (40 to 65 %)
13: Open (15 to 40 %)
15: Sparse (4 to 15 %)
16: Scattered (1 to 4 %)
Water Seasonality
The length of time an aquatic vegetated area was measured as being inundated
0: Not applicable (not an aquatic environment)
1: Semi-permanent or permanent (> 3 months)
2: Temporary or seasonal (< 3 months)
Water State
Describes whether the detected water is snow, ice or liquid water. Only liquid water is described in this release
0: Not applicable (not water)
1: Water
Intertidal
Delineates the intertidal zone
0: Not applicable (not intertidal)
3: Intertidal zone
Water Persistence
Describes the number of months a water body contains water
0: Not applicable (not an aquatic environment)
1: > 9 months
7: 7-9 months
8: 4-6 months
9: 1-3 months
Bare Gradation
Describes the percentage of bare in naturally bare areas
0: Not applicable (not a naturally bare area)
10: Sparsely vegetated (< 20 % bare)
12: Very sparsely vegetated (20 to 60 % bare)
15: Bare areas, unvegetated (> 60 % bare)
Level 4
All level 3 and level 4 classes for a given pixel are combined to give a single classification value
0: No data
1: Cultivated Terrestrial Vegetated
3: Cultivated Terrestrial Vegetated: Herbaceous
4: Cultivated Terrestrial Vegetated: Closed (> 65 %)
5: Cultivated Terrestrial Vegetated: Open (40 to 65 %)
6: Cultivated Terrestrial Vegetated: Open (15 to 40 %)
7: Cultivated Terrestrial Vegetated: Sparse (4 to 15 %)
8: Cultivated Terrestrial Vegetated: Scattered (1 to 4 %)
14: Cultivated Terrestrial Vegetated: Herbaceous Closed (> 65 %)
15: Cultivated Terrestrial Vegetated: Herbaceous Open (40 to 65 %)
16: Cultivated Terrestrial Vegetated: Herbaceous Open (15 to 40 %)
17: Cultivated Terrestrial Vegetated: Herbaceous Sparse (4 to 15 %)
18: Cultivated Terrestrial Vegetated: Herbaceous Scattered (1 to 4 %)
19: Natural Terrestrial Vegetated
20: Natural Terrestrial Vegetated: Woody
21: Natural Terrestrial Vegetated: Herbaceous
22: Natural Terrestrial Vegetated: Closed (> 65 %)
23: Natural Terrestrial Vegetated: Open (40 to 65 %)
24: Natural Terrestrial Vegetated: Open (15 to 40 %)
25: Natural Terrestrial Vegetated: Sparse (4 to 15 %)
26: Natural Terrestrial Vegetated: Scattered (1 to 4 %)
27: Natural Terrestrial Vegetated: Woody Closed (> 65 %)
28: Natural Terrestrial Vegetated: Woody Open (40 to 65 %)
29: Natural Terrestrial Vegetated: Woody Open (15 to 40 %)
30: Natural Terrestrial Vegetated: Woody Sparse (4 to 15 %)
31: Natural Terrestrial Vegetated: Woody Scattered (1 to 4 %)
32: Natural Terrestrial Vegetated: Herbaceous Closed (> 65 %)
33: Natural Terrestrial Vegetated: Herbaceous Open (40 to 65 %)
34: Natural Terrestrial Vegetated: Herbaceous Open (15 to 40 %)
35: Natural Terrestrial Vegetated: Herbaceous Sparse (4 to 15 %)
36: Natural Terrestrial Vegetated: Herbaceous Scattered (1 to 4 %)
55: Natural Aquatic Vegetated
56: Natural Aquatic Vegetated: Woody
57: Natural Aquatic Vegetated: Herbaceous
58: Natural Aquatic Vegetated: Closed (> 65 %)
59: Natural Aquatic Vegetated: Open (40 to 65 %)
60: Natural Aquatic Vegetated: Open (15 to 40 %)
61: Natural Aquatic Vegetated: Sparse (4 to 15 %)
62: Natural Aquatic Vegetated: Scattered (1 to 4 %)
63: Natural Aquatic Vegetated: Woody Closed (> 65 %)
64: Natural Aquatic Vegetated: Woody Closed (> 65 %) Water > 3 months (semi-) permanent
65: Natural Aquatic Vegetated: Woody Closed (> 65 %) Water < 3 months (temporary or seasonal)
66: Natural Aquatic Vegetated: Woody Open (40 to 65 %)
67: Natural Aquatic Vegetated: Woody Open (40 to 65 %) Water > 3 months (semi-) permanent
68: Natural Aquatic Vegetated: Woody Open (40 to 65 %) Water < 3 months (temporary or seasonal)
69: Natural Aquatic Vegetated: Woody Open (15 to 40 %)
70: Natural Aquatic Vegetated: Woody Open (15 to 40 %) Water > 3 months (semi-) permanent
71: Natural Aquatic Vegetated: Woody Open (15 to 40 %) Water < 3 months (temporary or seasonal)
72: Natural Aquatic Vegetated: Woody Sparse (4 to 15 %)
73: Natural Aquatic Vegetated: Woody Sparse (4 to 15 %) Water > 3 months (semi-) permanent
74: Natural Aquatic Vegetated: Woody Sparse (4 to 15 %) Water < 3 months (temporary or seasonal)
75: Natural Aquatic Vegetated: Woody Scattered (1 to 4 %)
76: Natural Aquatic Vegetated: Woody Scattered (1 to 4 %) Water > 3 months (semi-) permanent
77: Natural Aquatic Vegetated: Woody Scattered (1 to 4 %) Water < 3 months (temporary or seasonal)
78: Natural Aquatic Vegetated: Herbaceous Closed (> 65 %)
79: Natural Aquatic Vegetated: Herbaceous Closed (> 65 %) Water > 3 months (semi-) permanent
80: Natural Aquatic Vegetated: Herbaceous Closed (> 65 %) Water < 3 months (temporary or seasonal)
81: Natural Aquatic Vegetated: Herbaceous Open (40 to 65 %)
82: Natural Aquatic Vegetated: Herbaceous Open (40 to 65 %) Water > 3 months (semi-) permanent
83: Natural Aquatic Vegetated: Herbaceous Open (40 to 65 %) Water < 3 months (temporary or seasonal)
84: Natural Aquatic Vegetated: Herbaceous Open (15 to 40 %)
85: Natural Aquatic Vegetated: Herbaceous Open (15 to 40 %) Water > 3 months (semi-) permanent
86: Natural Aquatic Vegetated: Herbaceous Open (15 to 40 %) Water < 3 months (temporary or seasonal)
87: Natural Aquatic Vegetated: Herbaceous Sparse (4 to 15 %)
88: Natural Aquatic Vegetated: Herbaceous Sparse (4 to 15 %) Water > 3 months (semi-) permanent
89: Natural Aquatic Vegetated: Herbaceous Sparse (4 to 15 %) Water < 3 months (temporary or seasonal)
90: Natural Aquatic Vegetated: Herbaceous Scattered (1 to 4 %)
91: Natural Aquatic Vegetated: Herbaceous Scattered (1 to 4 %) Water > 3 months (semi-) permanent
92: Natural Aquatic Vegetated: Herbaceous Scattered (1 to 4 %) Water < 3 months (temporary or seasonal)
93: Artificial Surface
94: Natural Surface
95: Natural Surface: Sparsely vegetated
96: Natural Surface: Very sparsely vegetated
97: Natural Surface: Bare areas, unvegetated
98: Water
99: Water: (Water)
100: Water: (Water) Tidal area
101: Water: (Water) Perennial (> 9 months)
102: Water: (Water) Non-perennial (7 to 9 months)
103: Water: (Water) Non-perennial (4 to 6 months)
104: Water: (Water) Non-perennial (1 to 3 months)
Level 3 Class descriptions
Cultivated Terrestrial Vegetation (CTV)
Cultivated Terrestrial Vegetation (CTV) is associated with agricultural areas where active cultivation has been observed. In version 1.0 only herbaceous cultivation is shown and describes vegetation of strongly varying cover, ranging from bare (e.g. ploughed) areas to fully developed crops. Whilst the continental product describes land cover, interpretation is complicated as the same terminology is used to report on land use.
The definition of cultivated, and the difference to natural or semi-natural land covers, can be contentious particularly as much of the Australian landscape is used for agricultural food production. This includes areas of natural terrestrial vegetation (NTV) and natural aquatic vegetation (NAV) that are grazed by stock and which can be regarded as either semi-natural or cultivated.
CTV in the DEA Land Cover map is associated with areas where management practices aimed at cultivation (including for grass production) are actively performed during the year being shown. These practices include crop planting and harvesting, fertilization and ploughing. These practices often lead to highly dynamic spectral signals within and between years but also regular transitions between vegetation of different cover amounts as well as bare soil. This also means that agricultural areas will transition between natural and cultivated covers as management practices transition an area between actively cropped or grazed, to areas left fallow, areas reduced to low cover due to climate effects such as drought, or to other covers depending on what the predominant conditions are through the year being shown.
Natural Terrestrial Vegetation (NTV)
Natural Terrestrial Vegetation (NTV) represents areas that have all or most of the characteristics of natural or semi-natural herbaceous or woody vegetation (based primarily on floristics, structure, function and dynamics). These areas are identified as primarily vegetated, with either a photosynthetic vegetation fraction (PV) or non-photosynthetic fraction (NPV) greater than the bare soil fraction (BS) for at least two consecutive months. This approach considers that vegetation can exist in, and transition between, PV and NPV states during the year. In effect this approach classifies the landscape as primarily vegetated where the vegetated fraction of a pixel is greater than 30 %. Where the proportion of the landscape is under 30 % vegetated, it is regarded as sparsely vegetated or natural surface, but the cover proportions can still be quantified. Urban areas that are vegetated (e.g. suburbs with trees) are associated with NTV if the pixel is at least 30 % vegetated but artificial surfaces (AS) otherwise. The implementation allows areas of semi-natural vegetation (e.g. native grasslands/pastureland) to be included in the NTV class.
Natural Aquatic Vegetation (NAV)
Natural Aquatic Vegetation (NAV) is associated primarily with wetlands that are dominated by woody and/or herbaceous vegetation (as with NTV). NAV is generally associated with swamps, fens, flooded forests, saltmarshes or mangroves. Only mangroves are included in the current release.
Artificial Surfaces (AS)
Artificial Surfaces (AS) are areas of non-vegetated land cover created by human activities and are primarily represented by impervious surfaces (e.g. urban and industrial buildings, roads and railways). These can be more readily identified when the area is larger than the spatial resolution (25 m) provided by the sensor. Open cut extraction sites are often included in AS. However, there is considerable misclassification of NS as AS in areas where vegetated cover is very low and very consistent through the year.
Natural Surfaces (NS)
Natural Surfaces (NS) are comprised primarily of unconsolidated (often pervious, e.g. mudflats, saltpans) and/or consolidated (e.g. bare rock or bare soil) materials. In Australia, the proportional area of natural surfaces is relatively low and primarily confined to the deserts and semi-arid areas, river channels (e.g. dry riverbeds) and the coastline (e.g. sand dunes, mudflats). Much of the interior of Australia is sparsely vegetated and can be dominated by herbaceous (annual or perennial) or woody lifeforms.
Water
The Water class captures terrestrial and coastal open water such as dams, lakes, large rivers and the coastal and near-shore zone.
Level 4 Class Descriptions
Lifeform (NTV, NAV and CTV; 2 classes)
Lifeform represents the dominant vegetation type of a primarily vegetated area, discriminating woody from non-woody (herbaceous) vegetation. The Woody Cover Fraction models woody as vegetation of at least 2m in height and at least 20 % canopy cover. Hence the dominant vegetation in areas designated as woody in this product is considered to be composed of shrubs and trees. However where woody vegetation is not dominant in an area, the cover will be essentially herbaceous or bare. Hence some areas containing sparse trees or shrubs will likely be represented as herbaceous.
Vegetation Cover (NTV, NAV and CTV; 5 classes)
Vegetation cover is defined using the statistics of annual fractional cover of PV (for a calendar year). This relates to the upper-most foliage as observed from the Landsat satellite sensor, and describes the percentage of an area that is vegetated rather than bare.
Water Seasonality (NAV; 2 classes)
Water seasonality refers to the typical hydrological conditions in NAV within a year and is relevant to both coastal and inland wetlands. The current implementation utilises the Water Observations from Space (WOfS) dataset, identifying hydro-periods for NAV areas where water is (semi-) permanent (over 3 months) or temporary or seasonal (under 3 months).
Water State (Water; 1 class)
Water state establishes whether water is present in liquid form or as snow or ice. The current product only identifies areas where water is present as liquid for at least 20 % of observations (based on WOfS).
Water Persistence (Water; 4 classes)
Water persistence (or hydro-period) describes the maximum duration (in months) that water is seen to be covering the surface in the year.
Intertidal (Water; 1 class)
Intertidal water refers to primarily non-vegetated aquatic areas with systematic, tidal water variations.
Bare Gradation (NS; 3 classes)
The bare gradation describes the percentage of bare surface in areas which contain sporadic or little persistent green vegetation through the year. The percentage reflects that much of the remaining area is brown or dead vegetation and is characteristic of the more arid parts of Australia.
Lineage
The FAO LCCS taxonomy (Figure 1) is hierarchical and consists of a dichotomous phase (Level 1 to 3) and a modular phase (referred to as Level 4). In Level 1, vegetated and non-vegetated areas are first separated. These are then divided into terrestrial or aquatic categories to form Level 2. In the vegetated terrestrial category, cultivated and natural (including semi-natural) areas are differentiated. The non-vegetated category is further divided into artificial surfaces, and natural surfaces incorporating low vegetation cover and bare areas. Including the non-vegetated aquatic class (from Level 2), this results in the creation of six base land cover categories.
Figure 1 - Diagrammatic representation of the implementation of the FAO LCCS (Version 2) classification within the DEA Land Cover product version 1.0.
At Level 4, vegetated areas are further classified using information that differentiates lifeform (woody and herbaceous) and quantifies vegetation cover percent and water seasonality (for Natural Aquatic Vegetation). Natural Surface areas have information added (bare gradation) which describes the level of remaining vegetation present (sparse, very sparse or not detectable). Non-vegetated aquatic areas (Water) are further described on the basis of their persistence (hydroperiod) over a calendar year. The FAO LCCS differentiates water in different physical states (liquid or frozen; ice or snow), however only liquid water is included in the current release.
Figure 2 - Input data products used to produce Level 3 classification
Figure 3 - Input data products used to produce Level 4 classification
Software
References
Lucas R, Mueller N, Siggins A, Owers C, Clewley D, Bunting P, Kooymans C, Tissott B, Lewis B, Lymburner L, Metternicht G. Land Cover Mapping using Digital Earth Australia. Data. 2019; 4(4):143. https://doi.org/10.3390/data4040143
Christopher J. Owers, Richard M. Lucas, Daniel Clewley, Carole Planque, Suvarna Punalekar, Belle Tissott, Sean M. T. Chua, Pete Bunting, Norman Mueller & Graciela Metternicht (2021) Living Earth: Implementing national standardised land cover classification systems for Earth Observation in support of sustainable development, Big Earth Data, 5:3, 368-390, DOI: 10.1080/20964471.2021.1948179
Metternicht, G., Mueller, N., Lucas, R., Digital Earth for Sustainable Development Goals, Manual of Digital Earth, pp 443 - 471, Springer Singapore. https://doi.org/10.1007/978-981-32-9915-3_13
Accuracy
The product was validated using 6000 points spatially distributed over Australia. These points were created using a stratified random sampling approach slightly adjusted for oversampling. This process was conducted for 2010 and 2015 creating 12000 samples in total. After removing points with No Data and spurious values the total number was 11750. The sample points were divided into clusters for visual assessment against the outputs from the classification and assessed individually from a pool of 10 people. To compare the individual biases of the individual assessors, an additional set of validation points were created that all assessors evaluated, the results are shown in Table 4. Where assessors could identify a predominant land cover (i.e. not ‘mixed’ pixels or ‘unsure’), all assessors agreed 75 % of the time.
Table 2 contains the overall accuracy for all classes. The term ‘support’ refers to the number of validation points used in the calculation of that accuracy value.
Table 3 contains per-class accuracy information. “Precision” refers to the ability of a classification model to return only relevant instances. “Recall” refers to the ability to identify all relevant instances. The “F1 score” is a combination of precision and recall and an overall measure of accuracy. Classes such as artificial surfaces, natural aquatic vegetation and water had high accuracies. Classifying cultivated terrestrial vegetation and bare surfaces was challenging and accuracies were the lowest of the six classes presented here.
Limitations of the Implementation Method
DEA Land Cover is created by combining multiple layers that each describe features in the landscape. In doing so the extents of each layer do not necessarily completely align, and some no-data points can cross between outputs. As a result, there are some level 4 classes that only report detail to level 3 as the details of cover fraction and water persistence do not have corresponding data in the respective datasets. This specifically relates the classes of Water, NS and NAV in areas near water bodies and the intertidal zone, however the number of affected pixels is small.
Level 3 Class Limitations
Cultivated Terrestrial Vegetation (CTV)
Managed plantations and some orchards and tree crops are not currently distinguishable from semi-natural or natural terrestrial vegetation and are not yet incorporated in the area of CTV. Reference can be made to Australia’s National Plantation Inventory. In savanna regions (e.g. Queensland, Northern Territory and Western Australia), variable cycles associated with fires, inundation, drought and rainfall lead to greening or browning of natural vegetation that mirrors the seasonal or management-induced behavior of cultivated land. This leads to some areas of NTV, NS or NAV being misclassified as CTV. For example, the anomalous high levels of rainfall in 2010 led to vegetation growth patterns that were classified as cultivated vegetation, these false positives reduced the precision of the class in that year. Several natural vegetation types, particularly in the monsoonal north, are mapped as CTV due to burning, which can be associated with the indigenous management cycle. Saltmarsh and surface algae on mudflats can also be misclassified. Areas of bare soil exposed for long periods during the agricultural cycle or management activities, and sparse vegetation (when areas are not in use or fallow such as during drought periods) can be assigned as Natural Surfaces (NS). This is particularly the case where areas have experienced low cover for prolonged periods within a year.
Natural Terrestrial Vegetation (NTV)
The NTV category can transition into the NS category between years when a highly variable Landsat satellite spectral signature is observed due to changes in vegetation productivity and moisture content. The transition of NTV to NS is particularly evident when comparing periods with rainfall above (as in 2010) or below (e.g. drought; as in 2015) the average. Some confusion between surfaces that are primarily bare surfaces and non-photosynthetic vegetation remains and is also partly a function of the spectral reflectance of the underlying soil type. Vegetated areas shadowed by terrain can also be misclassified as non-vegetated. Areas of very low vegetation coverage are associated with the NS rather than NTV category, with the cover percentages shown as percentage bare. The assignment of vegetated suburbs to NTV is largely correct, but AS surfaces located beneath the canopy are not currently represented in the product. Some confusion between CTV and NTV can occur because of natural seasonal changes in native vegetation. The edges of salt lakes may be misclassified as NTV.
Natural Aquatic Vegetation (NAV)
Currently only mangroves are mapped in the NAV class. Other vegetated natural landscapes (e.g. saltmarsh, river red gum forests in the Murray Valley, surface algae and other inland wetlands) where water significantly influences edaphic conditions of substrate are not mapped. This is because, in the current implementation, the water mask is included to assist in the differentiation of vegetation and non-vegetation as the presence of water creates excess noise in the underlying Fractional Cover product. To reduce this noise, the WOfS product is used as a water mask in the Fractional Cover product, and hence it is unlikely to produce the combination of vegetation and water required for the NAV class.
Artificial Surfaces (AS)
Misclassification occurs with natural surfaces, particularly in the arid and semi-arid regions, open cut mine sites, salt lakes and pans, sand dunes and beaches. This is attributed to similarities in the variance of spectral signatures over a year. Misclassification occurs in some cultivated areas attributable to the predominance of sparse vegetation or when land is left fallow for most of the year. The current temporal variance mask is 250 m in spatial resolution, compared to the 25 m land cover product, resulting in artefacts appearing in the land cover from the masking process. In addition, urban areas with an area less than 250 m are often excluded. Cloud and data quality issues can lead to incorrect assignment of other land cover classes to AS such as in south-west Tasmania.
Natural Surfaces (NS)
Land used for agriculture may be associated with an NS class if ploughing or tillage has occurred and the vegetation cover remains low during the calendar year. Areas mapped as NS may, under certain circumstances (e.g. during drought) temporarily transition to or from CTV. This occurs because of the dominance of non-persistent vegetation (e.g. short crop life spans), desiccation, removal during dry periods or management practices (e.g. left as fallow). Where acquisition of cloud-free Landsat satellite sensor data has been infrequent, less information on the inter-annual variability of vegetation is provided. Where cloud cover is extensive (e.g. in Tasmania), the number of scenes for land cover classification is reduced and this can compromise classification of NS, particularly where bare surfaces alternate with vegetation. Confusion between natural surfaces and urban areas occurs because of similar spectral variation over an annual period.
Water
Areas of artificial and natural water are not differentiated, although the extent of the former is mapped within the existing Bureau of Meteorology Geofabric product. Due to the 25 m pixel size, rivers and water courses that cover less than a pixel, or with highly vegetated riverbanks are not captured, resulting in a patch-like representation of narrower rivers. Areas of dark, wet soil are often misclassified as water, including in cultivated areas and mud flats.
Level 4 Class Limitations
Lifeform
The woody discrimination is implemented using the Woody Cover Fraction product (Liao et al, 2020), which models woody cover from inputs of LiDAR including ICESat/GLAS, L-band SAR, field observation and Landsat satellite data. Issues arise in this dataset in areas dominated by short, woody vegetation such as heathland, and swampy regions where underlying water can introduce errors. Areas of woody savannah are also underrepresented due to the influence of the herbaceous understory dominating the observation.
Vegetation Cover
The cover of vegetation is derived from the fractional cover product (Scarth et al, 2010), and as such reflects the limitations of that product, mainly difficulty with measurement of non-photosynthetic vegetation, and noise due to the presence of water in a pixel. Thus arid areas can be difficult to fully analyse for cover, leading to misclassifications between NTV, NS and CTV where cover is sparse (lower than 15 %).
Water Seasonality
This product does not yet identify consecutive months but rather the frequency of wet observations in the year, based on the WOfS product. Therefore, monthly statements are unlikely to be consistent across the continent. Mangroves are currently the only consistently identified NAV and water cannot be easily observed beneath their canopies, which are often dense. Hence, the hydroperiod (and hence seasonality metrics) should be treated with some caution.
Water State
Mapping of ice and snow states has yet to be undertaken. Hence the water state class is currently limited to liquid only.
Intertidal
The intertidal areas carry the limitations of the ITEM product:
Intertidal areas are determined as a coastal mask, which currently identifies some non-tidal inland water bodies as tidal because of intra-annual changes in water extent.
This product is static and cannot be used to demonstrate change between two annual continental products.
Bare Gradation
Bare gradation is produced from the fractional cover product. Unlike the Vegetation Cover class, Bare Gradation is calculated from the median bare fraction, rather than consecutive, monthly green and non-photosynthetic fractions. Hence the bare fraction can be as low as 20 %, however this does not imply that the remaining fraction is healthy vegetation. Rather the remaining fraction is a mix of brown, dead and green vegetation, with intermittent green periods through the year, reflective of arid area vegetation types.
Earth Observation Limitations
To generate the land cover classification for each calendar year, annual (January – December) statistics derived from Landsat-5, -7 and -8 observations are currently used, with each satellite sensor potentially observing the Australian landscape every 16 days. This brings an intrinsic limitation to land cover mapping as persistent cloud in some regions reduces the number of useable observations. This is particularly evident in Tasmania, and northern Australia during the monsoon period, where areas may not be observed for extended periods and parts or all of the intra-annual land cover cycle may be missed. These limitations can lead to misclassifications of land cover, particularly in dynamic environments. In a future release, a confidence layer will be included to help identify areas with poor observation frequency or other factors impacting the classification.
An additional limitation of the Landsat series is the availability of data due to the ageing of each satellite. Landsat 5 was operational for over 25 years, but for much of the later years, data were only acquired when sunlight directly illuminated its solar panels. This limited its operation across Australia, with coverage being seasonally dependent, and contracting north to a minimum in winter. In its last years the winter coverage usually only covered the northern coasts of Australia. Landsat 5 ceased regular operations over Australia in 2011, leaving just Landsat 7 until Landsat 8 was launched in 2013. Landsat 7 began service in 1999 as a replacement for Landsat 5. Initially Landsat 5 was switched off, but when Landsat 7 suffered a serious problem in 2003 due to the failure of its scan-line corrector (termed SLC-Off) Landsat 5 resumed service. The SLC-Off failure of Landsat 7 results in severe striping across every image from mid 2003 onwards, apparent in subsequent derived products. Landsat 8 has operated well since launch in 2013, with improved sensitivity, noise characteristics and data correction in comparison to the earlier sensors.
The result of the availability of the satellites is that the most consistent data availability occurs when two satellites are in operation (most of the 2003 to present period). The least data availability is in 2011 – 2012 when only Landsat 7 was available with data containing the SLC-Off striping issue. The overall data availability for the Landsat satellites is shown in Table 5. The datasets used in this analysis are shown in Table 6.
Access the data
See it on a map |
Learn how to use DEA Maps |
|
Explore data availability |
Learn how to use the DEA Explorer |
|
Get the data online |
Learn how to access the data via AWS |
|
Code sample |
Learn how to use the DEA Sandbox |
|
Get via web service |
Learn how to use DEA’s web services |
Use constraints
DEA Land Cover is appropriate to use at the national scale where other more detailed information on land cover is not available. Where DEA land cover shows conflicting information to state or local datasets, those datasets should be considered authoritative.
How to view the data in a web map
To view and access the data interactively:
Visit DEA Maps.
Click
Explore map data
.Select
Land and vegetation
>DEA Land Cover
>DEA Land Cover (Landsat)
.Click
Add to the map
, or the+
symbol to add the data to the map.
How to load data with Python in the DEA Sandbox
DEA Sandbox allows you to explore DEA’s Earth Observation datasets in a JupyterLab environment. To sign up for DEA Sandbox see the user guide
Once you have access, click into the DEA products
directory to find the Introduction to DEA Land Cover
notebook on the Sandbox. This notebook will walk you through loading and visualising the DEA Land Cover data.
Downloading the DEA Landcover data
DEA Land Cover data can be downloaded from DEA’s public data holdings through a web browser, or using Amazon Web Service’s Command Line Interface (AWS CLI).
via web browser:
From here, simply navigate to the year and tile* of interest and directly download the GeoTIFF file for the layer you’re after.
To find x and y tile values for an area, see the Explorer here.
Bulk download using AWS CLI (for technical users):
First you need to install AWS CLI, instructions here
Then you can download data from the command line with a command such as:
aws s3 --no-sign-request sync s3://dea-public-data/derivative/ga_ls_landcover_class_cyear_2/1-0-0/2020 C:/landcover/ --exclude "*" --include "*_level4.tif"
(This downloads all level4 tiles for 2020 into a folder called ‘landcover’)
Basis of the command:
aws s3 --no-sign-request sync [1][2][3][4]
Where:
[1] The s3 bucket and folder to download data from: e.g.,
s3://dea-public-data/derivative/ga_ls_landcover_class_cyear_2/1-0-0/2020
[2] The directory to download to: e.g.,
C:/landcover/
[3] When you want to specify specific files to download, first you need to exclude everything
--exclude "*"
[4] Then you can define what you want to include (you can add this multiple times), e.g.,
--include "*_level4.tif" --include "*_level4_rgb.tif"
Adding DEA Landcover to QGIS
(for the time dimension to work you need version 3.22+)
From the drop down menus at the top select Layer
> Add Layer
> Add WMS/WMTS Layer
Click ‘New’ to setup a new data source, then enter
Name: DEA Services
URL: [https://ows.dea.ga.gov.au/](https://ows.dea.ga.gov.au/)
Click Connect
Once the items appear you can choose which layers to add.
Select Land and Vegetation
> DEA Land Cover
, then either:
DEA Land Cover Calendar Year (Landsat)
, then the basic or detailedDEA Land Cover Environmental Descriptors
, then any of the various descriptor layers (lifeform, water seasonality etc)
Once you have selected a layer, click Add
at the bottom of the window to add it to your project.
Temporal information can be accessed by clicking the clock icon next to the name of the layer in the layers list.
Adding DEA Landcover to ArcMap
First add Digital Earth Australia to the GIS Servers:
Select
Windows
>Catalog
>GIS Servers
>Add WMTS Server
Enter https://ows.dea.ga.gov.au/ into the URL field
Click
OK
Now add the layer to your map:
From the drop down menus at the top select
File
>Add Data
>Add Data...
Click the
Look in
selector, and chooseGIS Servers
Double click
Digital Earth Australia – OGC Web Services...
Select
DEA Land Cover Calendar Year (Landsat)
orDEA Land Cover Environmental Descriptors
Click
Add
Old versions
No old versions available.
Acknowledgments
DEA acknowledges the contribution of several collaborators who helped to create this Land Cover product:
CSIRO - Anna Richards, Becky Schmidt, Kristen Williams
UNSW - Graciela Metternicht
Department of Agriculture, Water and Environment - Jane Stewart, Lucy Randall, Lucy Gramenz
ANU - Albert van Dijk
Tasmania DPIPWE - Lindsay Mitchell
License and copyright
© Commonwealth of Australia (Geoscience Australia).
Released under Creative Commons Attribution 4.0 International Licence.