Displaying satellite imagery on a web map 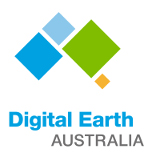
Sign up to the DEA Sandbox to run this notebook interactively from a browser
Compatibility: Notebook currently compatible with both the
NCI
andDEA Sandbox
environmentsProducts used: ga_s2bm_ard_3
Background
Leaflet is the leading open-source JavaScript library for mobile-friendly interactive maps. Functionality it provides is exposed to Python users by ipyleaflet and folium. This library enables interactive maps in the Jupyter notebook/JupyterLab environment.
Description
This notebook demonstrates how to plot an image and dataset footprints on a map.
Load some pixel data in
EPSG:3857
projection, same as used by most web mapsDisplay image loaded from these datasets on a map
Display dataset footprints on a map along with image loaded from these datasets on the same map
Getting started
To run this analysis, run all the cells in the notebook, starting with the “Load packages” cell.
Load packages
[1]:
import os
import ipyleaflet
import numpy as np
from ipywidgets import widgets as w
from IPython.display import display
import datacube
import odc.ui
from odc.ui import with_ui_cbk
import sys
sys.path.insert(1, '../Tools/')
from dea_tools.maps import folium_map, folium_dual_map
from dea_tools.maps import ipyleaflet_map
Connect to the datacube
[2]:
dc = datacube.Datacube(app='Imagery_on_web_map')
Find datasets
In this example we are using the Sentinel-2B ARD product. We will be visualizing a portion of the swath taken by Sentinel-2B on 13-Jan-2018.
We want to display the captured imagery, but later on we will also need the dataset footprints. Rather than calling dc.load(..)
directly with the time and spatial bounds we first use
dss = dc.find_datasets(..)
to obtain a list of datacube.Dataset
objects overlapping with our query first.
[3]:
# Define product and red/green/blue bands in the given product
product = 'ga_s2bm_ard_3'
RGB = ('nbart_red', 'nbart_green', 'nbart_blue')
# Region and time of interest
query = dict(
lat=(-30, -36),
lon=(137, 139),
time='2018-01-13',
)
dss = dc.find_datasets(product=product, **query)
print(f"Found {len(dss)} datasets")
Found 7 datasets
Load red/green/blue bands
Since we already have a list of datasets (dss
) we do not need to repeat the query, instead we supply datasets to dc.load(.., datasets=dss, ..)
along with other parameters used for loading data. Note that since we do not supply lat/lon
bounds we will get all the imagery referenced by the datasets found earlier and the result will not be clipped to a lat/lon
box in the query above.
We will load imagery at 200 m per pixel resolution (1/20 of the native) in the Pseudo-Mercator (EPSG:3857
) projection, same as used by most webmaps.
[4]:
rgb = dc.load(
product=product, # dc.load always needs product supplied, this needs to be fixed in `dc.load` code
datasets=dss, # Datasets we found earlier
measurements=RGB, # Only load red,green,blue bands
group_by='solar_day', # Fuse all datasets captured on the same day into one raster plane
output_crs='EPSG:3857', # Default projection used by Leaflet and most webmaps
resolution=(-200, 200), # 200m pixels (1/20 of the native)
resampling='bilinear', # Use bilinear resampling when scaling down
progress_cbk=with_ui_cbk()) # Display load progress
rgb
[4]:
<xarray.Dataset> Dimensions: (time: 1, y: 2436, x: 989) Coordinates: * time (time) datetime64[ns] 2018-01-13T00:57:00.462000 * y (y) float64 -3.478e+06 -3.478e+06 ... -3.965e+06 -3.965e+06 * x (x) float64 1.514e+07 1.514e+07 ... 1.534e+07 1.534e+07 spatial_ref int32 3857 Data variables: nbart_red (time, y, x) int16 -999 -999 -999 -999 ... -999 -999 -999 -999 nbart_green (time, y, x) int16 -999 -999 -999 -999 ... -999 -999 -999 -999 nbart_blue (time, y, x) int16 -999 -999 -999 -999 ... -999 -999 -999 -999 Attributes: crs: EPSG:3857 grid_mapping: spatial_ref
Place data on a map
We can display this data on a folium
map. We will use Datacube OWS styles to render the datasets into an image.
[5]:
# datacube OWS style configuration (see: https://datacube-ows.readthedocs.io/en/latest/styling_howto.html)
rgb_ows_cfg = {
"components": {
"red": {"nbart_red": 1.0},
"green": {"nbart_green": 1.0},
"blue": {"nbart_blue": 1.0},
},
"scale_range": (50, 3000),
}
folium_map(rgb, ows_style_config=rgb_ows_cfg, width=600, height=600)
[5]:
We can also use the ipyleaflet
version of this, even though we are not adding any custom interactivity to it here.
[6]:
ipyleaflet_map(rgb, ows_style_config=rgb_ows_cfg, layout=w.Layout(width='600px', height='600px'), scroll_wheel_zoom=True)
[6]:
Compare true color image with false color image
We are going to compare this image with a false color version that maps the Sentinel-2 SWIR-2 band to red, NIR-1 band to green, and the usual green band to blue.
[7]:
FALSE_RGB = ['nbart_swir_2', 'nbart_nir_1', 'nbart_green']
false_rgb_ows_cfg = {
"components": {
"red": {"nbart_swir_2": 1.0},
"green": {"nbart_nir_1": 1.0},
"blue": {"nbart_green": 1.0},
},
"scale_range": (50, 3000),
}
false_rgb = dc.load(
product=product, # dc.load always needs product supplied, this needs to be fixed in `dc.load` code
datasets=dss, # Datasets we found earlier
measurements=FALSE_RGB, # Only load red,green,blue bands
group_by='solar_day', # Fuse all datasets captured on the same day into one raster plane
output_crs='EPSG:3857', # Default projection used by Leaflet and most webmaps
resolution=(-200, 200), # 200m pixels (1/20 of the native)
resampling='bilinear', # Use bilinear resampling when scaling down
progress_cbk=with_ui_cbk()) # Display load progress
false_rgb
[7]:
<xarray.Dataset> Dimensions: (time: 1, y: 2436, x: 989) Coordinates: * time (time) datetime64[ns] 2018-01-13T00:57:00.462000 * y (y) float64 -3.478e+06 -3.478e+06 ... -3.965e+06 -3.965e+06 * x (x) float64 1.514e+07 1.514e+07 ... 1.534e+07 1.534e+07 spatial_ref int32 3857 Data variables: nbart_swir_2 (time, y, x) int16 -999 -999 -999 -999 ... -999 -999 -999 -999 nbart_nir_1 (time, y, x) int16 -999 -999 -999 -999 ... -999 -999 -999 -999 nbart_green (time, y, x) int16 -999 -999 -999 -999 ... -999 -999 -999 -999 Attributes: crs: EPSG:3857 grid_mapping: spatial_ref
The following gives us two maps showing the two datasets side-by-side that share the view controls for easy comparison.
[8]:
folium_dual_map(rgb, false_rgb, left_ows_style=rgb_ows_cfg, right_ows_style=false_rgb_ows_cfg, scroll_wheel_zoom=False)
[8]:
Create Leaflet Map with dataset footprints
This is a more advanced example with some interactivity (opacity control) supported by the ipyleaflet
package.
First we convert list of dataset objects into a GeoJSON of dataset footprints, while also computing bounding box. We will use the bounding box to set initial viewport of the map.
[9]:
polygons, bbox = odc.ui.dss_to_geojson(dss, bbox=True)
Create ipyleaflet.Map
with full-screen and layer visibility controls. Set initial view to be centered around dataset footprints. We will not be displaying the map just yet.
[10]:
zoom = odc.ui.zoom_from_bbox(bbox)
center = (bbox.bottom + bbox.top) * 0.5, (bbox.right + bbox.left) * 0.5
m = ipyleaflet.Map(
center=center,
zoom=zoom,
scroll_wheel_zoom=True, # Allow zoom with the mouse scroll wheel
layout=w.Layout(
width='600px', # Set Width of the map to 600 pixels, examples: "100%", "5em", "300px"
height='600px', # Set height of the map
))
# Add full-screen and layer visibility controls
m.add_control(ipyleaflet.FullScreenControl())
m.add_control(ipyleaflet.LayersControl())
Now we add footprints to the map.
[11]:
m.add_layer(ipyleaflet.GeoJSON(
data={'type': 'FeatureCollection',
'features': polygons},
style={
'opacity': 0.3, # Footprint outline opacity
'fillOpacity': 0 # Do not fill
},
hover_style={'color': 'tomato'}, # Style when hovering over footprint
name="Footprints" # Name of the Layer, used by Layer Control widget
))
Create Leaflet image layer
Under the hood mk_image_layer
will:
Convert 16-bit
rgb
xarray to an 8-bit RGBA imageEncode RGBA image as PNG data
odc.ui.to_rgba
Render PNG data to “data uri”
Compute image bounds
Construct
ipyleaflet.ImageLayer
with uri from step 3 and bounds from step 4
JPEG compression can also be used (e.g fmt="jpeg"
), useful for larger images to reduce notebook size in bytes (use quality=40
to reduce size further), downside is no opacity support unlike PNG.
Satellite imagery is often 12-bit and higher, but web images are usually 8-bit, hence we need to reduce bit-depth of the input imagery such that there are only 256 levels per color channel. This is where clamp
parameter comes in. In this case we use clamp=3000
. Input values of 3000
and higher will map to value 255
(largest possible 8-bit unsigned value), 0
will map to 0
and every other value in between will scale linearly.
[12]:
img_layer = odc.ui.mk_image_overlay(
rgb,
clamp=3000, # 3000 -- brightest pixel level
fmt='png') # "jpeg" is another option
# Add image layer to a map we created earlier
m.add_layer(img_layer)
Add opacity control
Add Vertical Slider to the map
Dragging slider down lowers opacity of the image layer
Use of
jslink
fromipywidgets
ensures that this interactive behaviour will work even on a pre-rendered notebook (i.e. on nbviewer)
[13]:
slider = w.FloatSlider(min=0, max=1, value=1, # Opacity is valid in [0,1] range
orientation='vertical', # Vertical slider is what we want
readout=False, # No need to show exact value
layout=w.Layout(width='2em')) # Fine tune display layout: make it thinner
# Connect slider value to opacity property of the Image Layer
w.jslink((slider, 'value'),
(img_layer, 'opacity') )
m.add_control(ipyleaflet.WidgetControl(widget=slider))
Finally display the map
[14]:
display(m)
Sharing notebooks online
Unlike notebooks with matplotlib
figures, saving a notebook after running it is not enough to have interactive maps displayed when sharing rendered notebooks online. You also need to make sure that “Widget State” is saved. In JupyterLab make sure that Save Widget State Automatically
setting is enabled. You can find it under Settings
menu.
Additional information
License: The code in this notebook is licensed under the Apache License, Version 2.0. Digital Earth Australia data is licensed under the Creative Commons by Attribution 4.0 license.
Contact: If you need assistance, please post a question on the Open Data Cube Slack channel or on the GIS Stack Exchange using the open-data-cube
tag (you can view previously asked questions here). If you would like to report an issue with this notebook, you can file one on
GitHub.
Last modified: September 2021
Compatible datacube version:
[15]:
print(datacube.__version__)
1.8.13
Tags
Tags: NCI compatible, sandbox compatible, sentinel 2, widgets, ipyleaflet, interactive